Introduction
Bioinformatics and biostatistics are two vital fields that contribute significantly to biological research and data analysis.
Bioinformatics primarily focuses on the application of computational tools and techniques to analyze and interpret complex biological data.
This field involves managing genomic, proteomic, and metabolomic information to derive meaningful insights.
Bioinformatics helps researchers understand biological processes by providing frameworks for data integration and visualization.
In contrast, biostatistics emphasizes the use of statistical methods to analyze data in health and biological sciences.
This discipline applies various statistical techniques to design studies, analyze experimental data, and interpret results.
Biostatisticians ensure that research findings are valid and reliable by employing rigorous statistical methodologies.
They also help establish the significance of findings through hypothesis testing and confidence intervals.
Understanding the key differences between bioinformatics and biostatistics is crucial for professionals in these interconnected fields.
Each discipline employs distinct methodologies, tools, and approaches tailored to specific research questions.
For example, bioinformatics often requires programming skills, familiarity with biological databases, and expertise in algorithms for analyzing high-throughput data.
Conversely, biostatistics relies heavily on statistical theory, models, and software to draw conclusions from data.
Definition and Scope of Bioinformatics
Computational Tools and Techniques
Bioinformatics primarily emphasizes using computational tools and techniques to analyze biological data.
It involves developing algorithms and software to process large-scale biological datasets.
Researchers in bioinformatics use programming languages like Python, R, and Java for data analysis.
They employ machine learning methods to identify patterns and trends in the data.
These tools help scientists manage the complexity of biological information effectively.
In contrast, biostatistics focuses on the application of statistical methods to biological and health-related data.
While biostatistics also utilizes computational tools, it emphasizes statistical theory and methodology.
Biostatisticians often design studies and analyze data to draw valid conclusions.
Their work primarily supports public health, clinical trials, and epidemiological research.
Applications in Genomics, Proteomics, and Systems Biology
Bioinformatics finds extensive applications in genomics, proteomics, and systems biology.
In genomics, bioinformatics helps analyze DNA sequences to identify genetic variations.
Researchers use bioinformatics tools to annotate genomes and study gene function.
This analysis plays a crucial role in personalized medicine, as it helps tailor treatments based on genetic information.
In proteomics, bioinformatics facilitates the analysis of protein structures and functions.
It helps researchers study protein interactions and post-translational modifications.
By integrating proteomic data with genomic information, bioinformatics enables a comprehensive understanding of cellular processes.
This approach is essential for discovering new drug targets and therapeutic strategies.
Systems biology is another area where bioinformatics excels.
Researchers use computational models to study complex biological systems.
Bioinformatics tools help simulate interactions among various biological components, such as genes, proteins, and metabolites.
This interdisciplinary approach fosters a holistic understanding of biological functions and their regulation.
Interdisciplinary Nature of Bioinformatics
Bioinformatics is inherently interdisciplinary, drawing from biology, computer science, mathematics, and statistics.
This fusion of disciplines enhances its ability to address complex biological questions.
For example, bioinformatics relies on computational algorithms to analyze data generated from high-throughput sequencing technologies.
It also requires a deep understanding of biological processes to interpret the results meaningfully.
The interdisciplinary nature of bioinformatics fosters collaboration among experts from diverse fields.
Biologists, computer scientists, and mathematicians work together to develop innovative solutions.
This collaborative environment encourages the exchange of ideas and knowledge, driving advancements in research.
Moreover, bioinformatics education emphasizes the importance of interdisciplinary training.
Aspiring bioinformaticians benefit from coursework in biology, programming, and statistics.
This broad skill set prepares them to tackle challenges in various biological domains.
Basically, bioinformatics and biostatistics serve distinct but complementary roles in biological research.
Bioinformatics focuses on computational tools and techniques to analyze biological data. Its applications span genomics, proteomics, and systems biology.
The interdisciplinary nature of bioinformatics fosters collaboration and innovation.
By understanding these key differences, researchers can effectively leverage the strengths of both fields to advance scientific knowledge.
Read: U.S. Cities with the Most Opportunities for Environmental Scientists
Definition and Scope of Biostatistics
The Use of Statistical Methods to Analyze and Interpret Biological Data
Biostatistics focuses on using statistical methods to analyze biological data.
It provides the framework for designing experiments and interpreting results.
Researchers apply biostatistics to make sense of complex data sets in biology and medicine.
Statistical methods help identify trends, patterns, and relationships within biological phenomena.
These methods enable scientists to draw valid conclusions from their research findings.
In biostatistics, data analysis is crucial for determining the significance of results.
Researchers often use various statistical techniques, including regression analysis and hypothesis testing.
These tools allow them to assess the impact of different variables on biological outcomes.
By employing robust statistical methods, biostatisticians ensure that their findings are reliable and meaningful.
Examples of Applications in Clinical Trials, Epidemiology, and Public Health
Biostatistics plays a vital role in clinical trials.
Researchers use statistical methods to design trials that test new treatments and interventions.
They calculate sample sizes, randomize subjects, and monitor outcomes.
Biostatisticians analyze trial data to evaluate the effectiveness of treatments.
This analysis informs decisions about whether to approve new drugs or therapies.
In epidemiology, biostatistics helps identify disease patterns and risk factors.
Researchers use statistical models to study the spread of diseases in populations.
By analyzing health data, they can identify trends and correlations.
This information is critical for public health interventions and policy decisions.
Public health also relies heavily on biostatistics for health surveillance.
Biostatisticians analyze data from various sources to monitor community health.
They help track disease outbreaks, vaccination rates, and health disparities.
Their analyses guide public health initiatives and resource allocation.
The Importance of Study Design and Data Analysis in Biostatistics
Study design is a fundamental aspect of biostatistics.
A well-designed study ensures that results are valid and applicable to real-world situations.
Researchers must carefully plan how to collect and analyze data before starting their investigations.
Proper study design minimizes bias and confounding factors.
Data analysis is equally important in biostatistics.
Researchers must choose appropriate statistical methods based on their study design and research questions.
This choice directly impacts the reliability of their findings.
Incorrect analysis can lead to misleading conclusions and ineffective interventions.
Moreover, biostatistics emphasizes the importance of reproducibility.
Other researchers should be able to replicate studies based on the original design and analysis methods.
This reproducibility strengthens the scientific foundation of biostatistical research.
Read: Environmental Scientist vs. Ecologist: Roles and Differences in the US
Educational Background and Training
Academic Paths and Requirements for Bioinformatics
To pursue a career in bioinformatics, students typically start with a strong foundation in biology and computer science.
A bachelor’s degree in bioinformatics, computational biology, or a related field is essential.
Some universities offer specialized bioinformatics programs that combine biology and computer science curricula.
Many aspiring bioinformaticians choose to further their education by obtaining a master‘s or doctoral degree.
Graduate programs often focus on advanced topics such as algorithms, data analysis, and molecular biology.
Students engage in hands-on projects that apply computational techniques to biological data.
In addition to formal education, bioinformatics professionals benefit from gaining experience through internships or research positions.
Practical experience helps them develop essential skills, including programming and data management.
Many employers prefer candidates with a solid background in programming languages like Python, R, or Java.
Strong Background in Computer Science, Biology, and Mathematics
A robust background in computer science is crucial for bioinformatics careers.
Professionals must be proficient in programming and algorithm design.
They often work with large datasets, requiring knowledge of data structures and software development.
A strong understanding of biology is also vital.
Bioinformaticians must comprehend biological concepts, including genetics, genomics, and molecular biology.
This knowledge allows them to analyze biological data accurately and draw meaningful conclusions.
Mathematics plays a significant role in bioinformatics as well.
Professionals should be comfortable with statistical methods and mathematical modeling.
These skills enable them to interpret data effectively and develop computational models.
Educational Requirements for Biostatistics
Biostatistics differs from bioinformatics in its focus on statistical analysis and research methods.
Students pursuing biostatistics typically start with a degree in statistics, mathematics, or public health.
Coursework usually includes statistical theory, biostatistics, and epidemiology.
Graduate programs in biostatistics emphasize advanced statistical methods and applications in public health research.
Students learn to design studies, analyze data, and interpret results.
Practical experience through internships or research projects is also essential for biostatistics professionals.
While both fields require a solid background in biology, the emphasis differs.
Biostatistics prioritizes statistics and epidemiology, while bioinformatics focuses on computer science and computational biology.
Therefore, both bioinformatics and biostatistics offer rewarding career paths in biological research.
Bioinformatics requires a strong foundation in computer science, biology, and mathematics.
Aspiring bioinformaticians should pursue degrees in bioinformatics or related fields and gain practical experience.
In contrast, biostatistics emphasizes coursework in statistics, biology, and epidemiology.
Students should pursue degrees that provide the necessary statistical and analytical skills.
Understanding these key differences helps aspiring professionals make informed decisions about their academic and career paths.
Read: The Relationship Between U.S. Policy & Environmental Scientist Roles
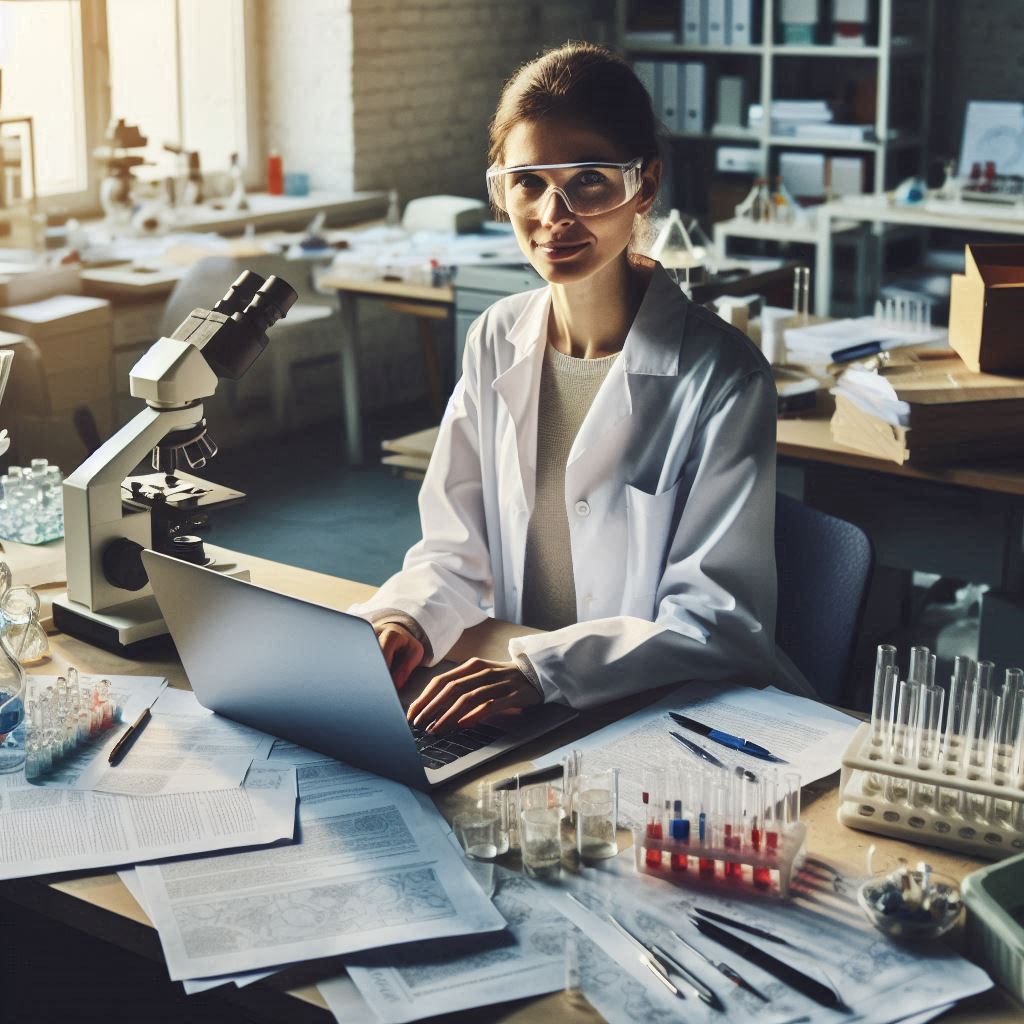
Research Focus and Objectives
The Primary Goals of Research in Bioinformatics
Research in bioinformatics primarily aims to analyze complex biological data.
One key goal is to identify patterns within large datasets.
Scientists use algorithms and statistical methods to reveal insights from genomic and proteomic data.
By recognizing these patterns, researchers can advance their understanding of biological processes.
Another significant objective in bioinformatics is predicting protein structures.
Accurate predictions help scientists understand protein functions and interactions.
Researchers utilize computational models and simulations to forecast how proteins fold and interact.
This information is crucial for drug discovery and therapeutic development.
Bioinformatics also focuses on modeling biological systems.
Scientists develop computational models to simulate biological processes and pathways.
These models help researchers explore interactions within cellular environments.
They can test hypotheses and predict how changes in one component affect the entire system.
This approach allows for a deeper understanding of complex biological phenomena.
Contrast with the Research Objectives in Biostatistics
In contrast, biostatistics emphasizes different research objectives.
One primary goal is to analyze the relationship between risk factors and disease outcomes.
Biostatisticians use statistical methods to identify correlations and causations.
This analysis helps in understanding how specific factors contribute to disease development.
Evaluating treatment effects is another vital objective in biostatistics.
Researchers design studies to assess the efficacy of medical interventions.
They analyze data from clinical trials to determine whether a treatment is effective.
This analysis guides medical decisions and shapes public health policies.
Biostatistics also focuses on designing experiments and surveys.
Researchers develop study protocols to ensure valid and reliable results.
They determine sample sizes, randomization methods, and data collection techniques.
Effective experimental design minimizes bias and enhances the credibility of findings.
While both fields utilize statistical methods, their goals and applications differ significantly.
Bioinformatics emphasizes computational analysis and modeling biological data.
Its primary focus is on understanding complex biological systems.
On the other hand, biostatistics prioritizes statistical analysis related to health outcomes and experimental design.
It aims to inform clinical practices and public health initiatives.
In fact, the primary goals of research in bioinformatics include identifying patterns, predicting protein structures, and modeling biological systems.
Conversely, biostatistics focuses on analyzing relationships between risk factors and diseases, evaluating treatment effects, and designing experiments.
Both fields contribute to scientific knowledge, but they approach biological questions from different perspectives.
Understanding these differences can help aspiring professionals choose their career paths effectively.
Read: Organizations & Associations for Environmental Scientists in the USA
Methodology and Tools
Computational Tools in Bioinformatics
Bioinformatics relies heavily on computational tools and algorithms.
Sequence alignment is a foundational technique used to compare DNA, RNA, or protein sequences.
It helps identify similarities and differences among biological sequences.
Common tools for sequence alignment include BLAST and Clustal Omega.
These tools aid in understanding evolutionary relationships and functional annotations.
Phylogenetic analysis is another critical area in bioinformatics.
It involves constructing evolutionary trees based on genetic data.
Researchers use algorithms like Neighbor-Joining and Maximum Likelihood to infer relationships between species.
These analyses reveal how different organisms are related and help trace evolutionary history.
Machine learning has gained popularity in bioinformatics recently.
Researchers apply machine learning algorithms to predict biological outcomes from large datasets.
Techniques like support vector machines and neural networks help classify data and discover patterns.
These approaches enhance drug discovery, genomics, and personalized medicine.
Statistical Methods in Biostatistics
In contrast, biostatistics employs various statistical methods to analyze biological data.
Regression analysis is a common technique that models relationships between variables.
It helps researchers understand how different factors influence health outcomes.
Linear and logistic regression are widely used in biostatistics.
Survival analysis is another important method.
It focuses on time-to-event data, commonly used in clinical studies.
Researchers analyze survival times to assess the effectiveness of treatments or interventions.
Techniques like the Kaplan-Meier estimator and Cox proportional hazards model are fundamental in this area.
Meta-analysis is a powerful biostatistical tool that combines results from multiple studies.
This technique enhances the reliability of findings by increasing sample size and statistical power.
Researchers use meta-analysis to draw broader conclusions about health interventions and outcomes.
Collaboration Between Bioinformaticians and Biostatisticians
The differences in tools and methods highlight the importance of collaboration between bioinformaticians and biostatisticians.
Interdisciplinary research projects benefit from integrating bioinformatics and biostatistics.
For example, bioinformaticians can generate large datasets from genomic studies.
Meanwhile, biostatisticians can apply statistical methods to analyze these datasets effectively.
Collaboration fosters a comprehensive understanding of biological questions.
Bioinformaticians provide insights into data generation and analysis, while biostatisticians ensure robust statistical interpretations.
Together, they can tackle complex research problems that neither discipline could solve alone.
Furthermore, joint efforts can lead to the development of new methodologies.
For instance, integrating machine learning techniques with traditional statistical methods can enhance predictive models.
This synergy paves the way for innovative solutions in personalized medicine, public health, and epidemiology.
Bioinformatics and biostatistics play vital roles in biological research.
They utilize different computational tools and statistical methods to analyze data.
Understanding their key differences can foster collaboration between these fields.
Together, bioinformaticians and biostatisticians can drive advancements in science and improve health outcomes.
Their partnership is essential in addressing the complexities of modern biological research.
Transform Your Career Today
Unlock a personalized career strategy that drives real results. Get tailored advice and a roadmap designed just for you.
Start NowCareer Opportunities and Job Prospects
Overview of Diverse Career Paths in Bioinformatics
Bioinformatics offers a wide array of career paths for professionals.
These roles span various sectors, including academia, industry, healthcare, and government.
In academia, bioinformaticians often conduct research and teach at universities.
They collaborate on innovative projects, pushing the boundaries of biological knowledge.
In industry, bioinformatics professionals work in pharmaceuticals and biotechnology companies.
They analyze data to support drug discovery and development processes.
Their work helps improve the efficacy of therapies and diagnostics.
In healthcare, bioinformatics experts manage and analyze genomic data.
They play a crucial role in personalized medicine and patient care.
Government positions also exist in bioinformatics.
Professionals in these roles often focus on public health and policy.
They analyze data to inform health decisions and regulations.
Overall, bioinformatics professionals can choose from diverse career opportunities tailored to their interests.
Growing Demand for Skilled Biostatisticians
Biostatistics, a related field, is experiencing a surge in demand.
Skilled biostatisticians are increasingly sought after in pharmaceuticals and biotechnology.
Companies rely on their expertise to analyze clinical trial data.
This analysis ensures the safety and efficacy of new drugs.
Public health organizations also seek biostatisticians.
They utilize statistical methods to study health trends and disease outbreaks.
Biostatisticians help design studies that inform health policies and interventions.
Environmental science is another area where biostatisticians contribute.
They analyze data related to environmental factors impacting public health.
The growing emphasis on data-driven decision-making fuels this demand.
Organizations across various sectors require skilled professionals to interpret complex datasets.
As a result, biostatistics has become an essential discipline in contemporary research.
Potential for Career Advancement and Competitive Salaries
Both bioinformatics and biostatistics offer promising career advancement opportunities.
Professionals in these fields can move into senior positions or management roles.
With experience, they can lead research teams or projects.
Advanced degrees, such as a Ph.D., can further enhance career prospects.
In addition to advancement opportunities, both fields offer competitive salaries.
Bioinformatics professionals earn attractive salaries, especially in industry roles.
Their skills are in high demand, reflecting their specialized training.
Similarly, biostatisticians enjoy competitive compensation due to their expertise.
Organizations recognize the value of skilled professionals who can drive research forward.
Moreover, continuous learning opportunities exist in both fields.
Professionals can attend workshops, conferences, and training programs to enhance their skills.
This commitment to lifelong learning can lead to further career growth.
In essence, both bioinformatics and biostatistics present exciting career paths.
Bioinformatics professionals can work in various sectors, while biostatisticians are increasingly needed across industries.
The growing demand for skilled professionals ensures job security and competitive salaries.
As these fields evolve, the potential for career advancement continues to expand.
Aspiring professionals should consider the diverse opportunities available in bioinformatics and biostatistics to shape their careers.
Challenges and Ethical Considerations
Ethical Issues in Bioinformatics
Handling and interpreting biological data in bioinformatics raises several ethical issues.
Data privacy is a significant concern, especially with sensitive genetic information.
Protecting personal data from unauthorized access is paramount.
Researchers must implement stringent data security measures to ensure confidentiality.
Informed consent is another critical ethical issue.
Participants in studies must understand how their data will be used.
Researchers should provide clear information about data collection and potential risks.
Obtaining informed consent builds trust and ensures ethical compliance in bioinformatics research.
Data sharing poses further ethical challenges.
While sharing data promotes collaboration, it also raises concerns about ownership and privacy.
Researchers must balance the benefits of sharing with the need to protect individual privacy.
Establishing guidelines for responsible data sharing can help mitigate these risks.
Challenges in Biostatistics
Biostatistics also faces unique challenges in designing experiments and interpreting results.
One major challenge is bias, which can skew results and lead to incorrect conclusions.
Researchers must carefully design studies to minimize bias.
Randomization and blinding are effective strategies to reduce its impact.
Confounding factors complicate the interpretation of statistical results.
These factors can create false associations between variables.
Researchers must identify and control for confounding variables during analysis.
Failure to address these factors can lead to misleading conclusions.
Sample size is another critical consideration in biostatistics.
An inadequate sample size can reduce the power of a study, making it harder to detect significant effects.
Researchers should conduct power analyses before beginning experiments to determine the necessary sample size.
This ensures that the study has enough statistical power to yield reliable results.
The Need for Rigorous Validation and Replication
Both bioinformatics and biostatistics emphasize the need for rigorous validation and replication of research findings.
Validating results ensures that the findings are reliable and reproducible.
Researchers should use multiple datasets to confirm their findings and strengthen conclusions.
Replication is vital in both fields to establish credibility.
Successful replication of studies fosters confidence in research outcomes.
It also helps identify potential errors or biases in the original studies.
Ultimately, bioinformatics and biostatistics play essential roles in analyzing biological data.
Ethical issues, such as data privacy and informed consent, are crucial in bioinformatics.
In contrast, biostatistics grapples with challenges like bias, confounding factors, and sample size.
Both fields must prioritize rigorous validation and replication to ensure the integrity of research findings.
Understanding these differences and challenges can guide professionals in navigating their respective fields effectively.
Gain More Insights: Online Courses for Nanotechnology Enthusiasts
Conclusion
After examining the distinctions between bioinformatics and biostatistics, it is evident that these fields operate at the intersection of biology and data analysis.
Bioinformatics emphasizes the use of computational tools to analyze biological data, while biostatistics focuses on applying statistical methods to interpret health-related information.
Both fields play crucial roles in advancing our understanding of complex biological systems and facilitating evidence-based decision-making in healthcare.
It is essential for researchers and practitioners in these domains to recognize the unique opportunities and challenges each field presents.
By collaborating and engaging in interdisciplinary research, scientists can leverage the strengths of bioinformatics and biostatistics to drive innovation and discovery in the biological sciences.
Moving forward, the integration of diverse perspectives and methodologies will be key to addressing complex biological questions and improving human health outcomes.
In closing, bioinformatics and biostatistics offer distinct yet complementary approaches to analyzing biological data and advancing our understanding of the natural world.
Readers are encouraged to explore the opportunities in each field and consider the importance of collaboration in driving scientific progress.
Ultimately, collaboration and interdisciplinary research are essential in harnessing the full potential of bioinformatics and biostatistics to expand knowledge and catalyze innovation in the biological sciences.
[E-Books for Sale]
The Big Book of 500 High-Paying Jobs in America: Unlock Your Earning Potential
$19.99 • 500 High-Paying Jobs • 330 pages
Explore 500 high-paying jobs in America and learn how to boost your career, earn more, and achieve success!
See All 500 High-Paying Jobs of this E-Book
1001 Professions Without a Degree: High-Paying American Jobs You Can Start Now
$19.99 • 1001 Professions Without a Degree • 174 pages
Discover 1001 high-paying jobs without a degree! Unlock career tips, skills, and success strategies for just $19.99!